This article has been:Browse 1369Times Download 1022Times |
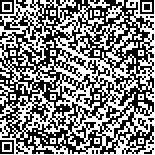 scan it! |
|
DOI:10.13522/j.cnki.ggps.20180198 |
|
Predicting Monthly Rainfall Using the Grey-correlation Method |
SUN Xiaoting, REN Ganghong, DU Kun, FENG Yan, ZHOU Ming
|
Faculty of Civil Engineering and Mechanics, Kunming University of Science and Technology, Kunming 650500, China
|
Abstract: |
【Objective】 Precipitation is the main input to many catchments and ecosystems, and catchment management and design of irrigation schedule need to predict it. This paper presents a method to predict monthly precipitation. 【Method】 Considering that temporal change of monthly precipitation is nonlinear and multi-scale, this paper proposed a data-driven based fusion method, in which the grey model (EGM) and the adaptive neuro-fuzzy inference system (ANFIS) were used to predict the rainfall at yearly and monthly scales. The two predictions were then fused by the grey correlation method. The model was evaluated against the rainfall data collected from eight sites in Vitoria of Australia. We also compared the proposed model with the so-called sole prediction models which include EGM (1,1), ANFIS, artificial neural network (ANN) and the autoregressive integrated moving average (ARIMA). 【Result】 The proposed model was more accurate than EGM(1,1), ANFIS and ANN and ARIMA model, giving the most accurate predication for five out of the eight sites, in which the root mean square errors of the proposed model for the central region (sites at Ballarat and Cape Otway) and the eastern region (Dookie, Wangaratta and Orbost stations) was 28.2 to 37.2 mm respectively, while for the western region (Dimboola, Edenhope and Dunkeld sites) it was 20.8 to 37.2 mm. 【Conclusion】 The proposed model is adequate and reliable, offering an alternative to predict monthly precipitation. |
Key words: monthly rainfall prediction; data-driven; model fusion; adaptive fuzzy neural network system; grey prediction model |
|
|