This article has been:Browse 677Times Download 419Times |
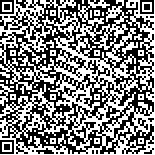 scan it! |
|
DOI:10.13522/j.cnki.ggps.2022222 |
|
Sustainable Agricultural Resource Utilization in Northwestern China Determined Using the Wavelet-PSOSVM |
WANG Chenxuan, CHEN Li, ZHANG An’an
|
1. School of Economics and Management, Xiamen University of Technology, Xiamen 361024, China;
2. School of Economics and Management, Anhui Jianzhu University, Hefei 230601, China
|
Abstract: |
【Objective】The initiative of “Western Development” launched by Chinese Government in 1999 had greatly benefited the northwestern provinces in the country including Shaanxi, Gansu, Ningxia and Xinjiang. As ecological systems in these provinces are fragile, rational use of agricultural resources is crucial to their sustainable development. The purpose of this paper is to present a method to help sustainable use of their agricultural resources. 【Method】The method is based on the wavelet-PSOSVM (particle swarm optimization (PSO) and support vector machine techniques (SVM)). The evaluation indexes are constructed using data measured from 2018 to 2020 in six aspects: economy, science and technology, society, natural environment, resources, and ecological governance. 【Result】①The mean square error and the correlation coefficient of the wavelet-PSOSVM are 9.411 5×10-5 and 0.968, respectively. In contrast, the mean square error and the correlation coefficient of PSOSVM are 0.015 3 and 0.967, respectively, using the same training and test set. The wavelet process improves the predicting accuracy and convergence of the PSOSVM. ②The mean square error and the correlation coefficient of the wavelet-SVM are 20.836 and 0.748 respectively, while the mean square error and correlation coefficient of the SVM are 30.903 and 0.634, respectively, using the same training and test sets. The wavelet process also improvs the predicting accuracy and convergence of the SVM.【Conclusion】Using wavelet process in both PSOSVM and SVM algorithms significantly improves their prediction accuracy, convergence, and training time efficiency, while reducing their complexity. Its application for evaluating sustainable utilization of agricultural resources in the four provinces in northwestern China indicates that, in terms of sustainability, Xinjiang comes to the top, followed by Gansu, with Shaanxi and Ningxia ranked in third and fourth, respectively. |
Key words: wavelet analysis; particle swarm optimization; support vector machine; resource sustainability utilization assessment |
|
|