This article has been:Browse 577Times Download 1165Times |
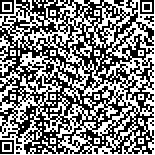 scan it! |
|
DOI:10.13522/j.cnki.ggps.2022588 |
|
Using Artificial Neural Network to Solve Multi-objective Optimization of Francis Turbine Runner |
WANG Rongtao, LAI Xide, CHEN Xiaoming
|
Xihua University, School of Energy and Power Engineering, Chengdu 610039, China)
|
Abstract: |
【Background】Technical renovation of turbine equipment in hydropower plant has attracted increased attention due to its low investment, quick return and high economic benefit. The purpose of modifying turbine equipment in hydropower station is to increase its capacity or improve its operation performance. Generally, it is impossible to make large modification to embed components in turbine modification. Most modifications aimed at the runners or the guide vanes. It is important to study effective multi-objective runner optimization design method to ensure efficiency, cavitation performance and hydraulic stability of the unit, as well as the comprehensive performance of the unit under different operating conditions.【Objective】This paper is to explore an optimization method which can simultaneously improve the operation efficiency, cavitation performance and operation stability of the Francis turbine, and provide a technical approach for multi-objective optimization of the Francis turbine runner.【Method】Taking the position angle and installation angle of runner blade inlet and outlet as optimization variables, the sample database was obtained by random discrete sampling of blade geometry parameters. The performance parameters of each sample were obtained based on CFD numerical calculation. A multi-objective function was then established, considering runner efficiency, swirl numbers at the outlet and cavitation coefficient of the Francis turbine. The mapping relationship between optimization variables and the multi-objective function is established based on artificial neural network. Runner blades with18 geometric parameters are optimized by genetic algorithm, and the performance of the runner blades before and after optimization is compared and analyzed. 【Result】For guide vane opening 112 degrees with running head being 160 m, 175 m or 180 m, the optimized runner efficiency was increased by 0.22%, 0.56% and 0.60% respectively compared with that without optimization. The optimization also improved the pressure distribution in the blades, and reduced pressure fluctuation amplitude at the vaneless area of the runner and the conical section of the draft tube.【Conclusion】The greater the optimized blade inlet placement angle was, the greater the improvement of comprehensive performance of Francis turbine would be. |
Key words: francis turbine; runner; multi-objective optimization design; artificial neural network; genetic algorithm |
|
|