This article has been:Browse 458Times Download 358Times |
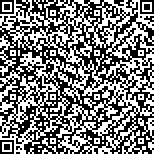 scan it! |
|
DOI:10.13522/j.cnki.ggps.2023047 |
|
Construction of Knowledge Graph Model for Irrigation Water Scheduling |
SU Nan, ZHANG Shaohui, BAI Meijian, ZHANG Baozhong
|
1. China Institute of Water Resources and Hydropower Research, Beijing 100038, China;
2. State Key Laboratory of Simulation and Regulation of Water Cycle in River Basin, Beijing 100038, China
|
Abstract: |
【Background and Objective】The adoption of artificial intelligence technology, particularly through adaptive learning, is reshaping the landscape of intelligent construction in the field of irrigation. This innovative perspective is divergent from traditional research paradigms, as numerous intelligent algorithms and technologies have been employed in the realm of irrigation water dispatching decisions. However, existing results often lack user-friendliness and ease of operation, preventing them from practical implementation. In light of this, this paper undertakes a comparative analysis of the Random Forest model and the BP Neural Network model in artificial intelligence technology.【Method】Building on this insight, the study divides historical data, based on measured values, into various gradients. These gradients encapsulate water irrigation scheduling variables, resulting in 9.82×1011 water dispatching scenarios. A pre-trained Random Forest model is employed to predict the dispatching flow corresponding to each scenario, ultimately constructing a fundamental database mapping the relationship between irrigation area water dispatching scenarios and dispatching flow values. This process enriches and encodes the scheduling scenario database through the utilization of machine learning models and existing scheduling history data. Leveraging this scenario database, and Neo4j technology, a knowledge map-based water dispatching flow prediction model is established for the Pishihang irrigation district.【Result and Conclusion】The key advantage of this model is its user-friendly approach. Irrigation area water scheduling managers can effortlessly determine the approximate gradient value of each scheduling variable within the knowledge graph model for a specific scheduling scenario. By doing so, they can readily access the corresponding scheduling flow prediction value through a simple query. Application results demonstrate that the knowledge map-based scheduling flow prediction model boasts a remarkable accuracy within the cognitive range of Pishihang irrigation district water management, facilitating real-time scheduling flow inquiries. This research represents a significant step toward more user-friendly and effective irrigation water management. |
Key words: Pishihang Irrigation District; water dispatching; machine learning; SHAP method; knowledge graph |
|
|