This article has been:Browse 438Times Download 341Times |
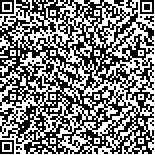 scan it! |
|
DOI:10.13522/j.cnki.ggps.2022653 |
|
Comparison of Three Models for Predicting Water Quality in Mishan Reservoir |
HUANG Linxian, ZHANG Mingfang, QIAN Yong, XING Xuerui, XING Liting, HAN Zhong
|
1. School of Water Conservancy and Environment, University of Jinan, Jinan 250022, China; 2. Weihai Hydrological Center,
Weihai 264209, China; 3. Institute of Hydrogeology and Environmental Geology, Chinese Academy of Geological Sciences, Shijiazhuang 050061, China; 4. Key Laboratory of Groundwater Remediation of Hebei Province and China Geological Survey, Shijiazhuang 050061, China; 5. Shandong Zhengyuan Geological Resources Exploration Co. Ltd., Jinan 250101, China;
6. No.6 Institution of Geology and Mineral Resources Exploration of Shandong Province, Weihai 264209, China
|
Abstract: |
【Objective】Accurate prediction of water quality is of great importance for protecting water ecological environment and improving water resource management, but difficult due to the combined influence of various factors including human activities, rainfall, temperature and hydrodynamic conditions which are complex and uncertain. In this paper we compare three models, the SARIMA model, Holt-Winters model and LSTM neural network model, for predicting water quality of reservoir.【Method】Electric conductivity of water was used as a proxy for water quality. Data measured monthly from 2012—2018 from Mishan Reservoir was used for model training, and the data measured in 2019 was used to test the models.【Result】The SARIMA and Holt-Winters models are comparatively simple, but because they only consider time series of water quality data, their accuracy is low. In contrast, the LSTM neural network model considers factors that affect water quality and implicitly represents the nonlinearity of the factors in their impact on water quality, it is more accurate than other two models.【Conclusion】In general, the LSTM neural network model is reliably, giving rise to large error only when there were sudden changes in the conductivity. Overall, it is reliable and accurate for predicting change in water quality induced by variations in the environment. |
Key words: time series model; LSTM model; conductivity; water quality prediction; Mishan Reservoir |
|
|