This article has been:Browse 602Times Download 300Times |
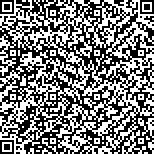 scan it! |
|
DOI:10.13522/j.cnki.ggps.2023251 |
|
Extracting Crop Structure from Remote Sensing Images of Qingtongxia Irrigation District Using the Threshold Feature-machine Learning Method |
YU Wuyang, WANG Yibo, CHEN Xinguo, HUANG Quanzhong, HUANG Guanhua
|
1. College of Water Resources and Civil Engineering, China Agricultural University, Beijing 100083, China;
2. Center for Agricultural Water Research in China, China Agricultural University, Beijing 100083, China;
3. Chinese-Israeli International Center for Research and Training in Agriculture, Beijing 100083, China
|
Abstract: |
【Objective】Tracking the change in cultivation areas of different crops in a basin or catchment is essential for agricultural management but challenging. The objective of this paper is to investigate the feasibility of threshold feature - random forest classification model for extracting crop structure from remote sensing imageries of irrigation district. We took Qingtongxia irrigation as an example.【Method】Using field survey and visual interpretation, the threshold feature-random forest classification method in the Google Earth Engine platform was used to delineate the planting areas of spring wheat, spring maize, and rice within the Qingtongxia irrigation district from 2013 to 2020.【Result】The threshold feature-random forest classification method is effective for extracting planting structure in the Qingtongxia irrigation district in the seven years, with an overall accuracy of 0.88 and a Kappa coefficient of 0.76. The linear fitting between the extracted areas from the remote sense images and areas obtained from survey gave a R2 which was 0.80, 0.93 and 0.86, for spring wheat, spring maize and rice, respectively. Data analysis revealed that the planting area of spring maize surpassed that of rice and spring wheat in 2013 to 2020. Spring maize cultivation had notably increased, while spring wheat cultivation initially increased and then declined; rice cultivation also decreased. Spring maize was mainly grown in the North and South of the irrigation district, while the overall irrigation area increased from the North to the South. Spring wheat was primarily cultivated in central and Southern parts of the irrigation district, and rice was in the central region. Spring wheat and rice cultivation areas increased from the South to the North.【Conclusion】The threshold feature-random forest classification model is effective and accurate for extracting annual change in planting structures in irrigation districts in arid regions. It is novel and perspective for understanding long-term evolution in planting structure in a region. |
Key words: crop structure; characteristic index; random forest; Qingtongxia irrigation district; grain crop |
|
|