This article has been:Browse 1310Times Download 2572Times |
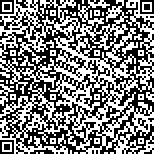 scan it! |
|
DOI:10.13522/j.cnki.ggps.2021385 |
|
Predicting Monthly Precipitation Using Chaotic Model |
SHU Tao, LU Haotian, CAO Jingxuan, et al.
|
1.College of Marine Geosciences, Ocean University of China, Qingdao 266100, China;
2. College of Earth Sciences, Guilin University of Technology, Guilin 541004, China;
3.College of Engineering, Tibet University, Lhasa 850000, China; 4. Department of Construction Engineering,
Dalian University of Technology, Dalian 116024, China; 5. Key Laboratory of Mountain Hazards and Earth Surface Process,
Chinese Academy of Sciences, Chengdu 610041, China; 6. Institute of Mountain Hazards and Environment,
Chinese Academy of Sciences & Ministry of Water Conservancy, Chengdu 610041, China;
7.University of Chinese Academy of Sciences, Beijing 100049, China
|
Abstract: |
【Objective】The time series of rainfall is a nonlinear, non-stationary process and can be analyzed statistically. The purpose of this paper is to analyze the chaotic characteristics of rainfalls in attempts to develop a chaotic model to predict monthly precipitation.【Method】We took monthly precipitation measured from the weather station at Bomi between Linzhi and Bomi on the G318 highway as an example, the C-C correlation integral method was used to determine the delay time t and the embedding dimension m in it. The time series was then reconstructed in phase space whose Lyapunov exponent was obtained for a small sub-dataset to determine the chaotic characteristics, from which we constructed a Volterra model to predict monthly rainfall in both short-term (5 years) and long-term (15 years) respectively. The predicted monthly rainfalls using the proposed model were compared with those predicted by the wavelet model and the SVR prediction model.【Result】The MAPE and EC of the rainfalls predicted using the proposed Volterra model for short-term was 4.04% and 0.941 respectively. Compared with the wavelet and SVR model, the proposed Volterra model was more accurate, and its superposition prediction error was smaller, with its associated MAPE and EC being 7.657% and 0.894 respectively. However, the rainfalls predicted by the proposed for long-term were not as good as those by the SVR model. When the time series of the rainfall was less chaotic, the prediction of Volterra model for short-term rainfall was less reliable, with its associated MAPE and EC being 54.855% and 0.566, respectively.【Conclusion】The chaotic model was more accurate than the traditional model for predicting monthly rainfall only for short-term and when the time series of the rainfalls is chaotic. Therefore, it should be used with care. |
Key words: chaotic model; phase space reconstruction; lyapunov index; volterra filter; rainfall prediction |
|
|