This article has been:Browse 1831Times Download 3599Times |
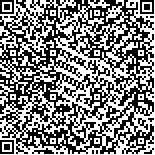 scan it! |
|
DOI:10.13522/j.cnki.ggps.20180410 |
|
Using Neural Network Model to Simplify ET0 Calculation for Representative Stations in Guangdong Province |
ZHAO Wengang, MA Xiaoyi, LIU Xiaoqun, SHI Lin, SONG Wen
|
1. Hunan Water Resources and Hydropower Research Institute, Changsha 410007, China; 2. Key laboratory of Agricultural Soil and Water Engineering in Arid and Semiarid Areas, College of Water Resources and Architectural Engineering Northwest A&F University, Yangling 712100, China
|
Abstract: |
【Objective】The purpose of this paper to investigate the feasibility of using BP, wavelet neural network and learning machine algorithm to differentiate the factors which affect the evapotranspiration at representative stations in Guangdong province. 【Method】We selected stations at Shaoguan, Shenzhen, Guangzhou, Jiexi and Zhanjiang as examples; and analyzed daily verage temperature, the highest and the lowest temperature, relative humidity, sunshine duration and wind speed, measured from 1981 to 2010 in these stations. The optimal estimation was determined by comparing the ET0 estimated using the FAO-56 penman Monteith with those calculated using the above three methods. The simplified model for ET0 was then established using factor sensitivity analysis. 【Result】①The meteorological factors in Guangzhou and Shaoguan differed significantly at P=0.05 level. Apart from daily maximum temperature, other meteorological factors in Zhanjiang, Guangzhou, Jiexi and Shenzhen did not show significant difference. ②The factor sensitivity analysis revealed that ET0 at Shaoguan, Guangzhou and Shenzhen was sensitive to the lowest and the highest daily temperature and the sunshine hours, with the sensitive coefficient associated with the three factors for Shaoguan being 0.040, 0.113 and 0.223, for Guangzhou being 0.043, 0.101 and 0.208, and for Shenzhen being 0.054, 0.105 and 0.18. ET0 at Jiexi and Zhanjiang was most sensitive to the highest temperature, relative humidity and sunshine duration, with the sensitive coefficient associated with them being 0.105, -0.040 and 0.216 for Jiexi, and 0.098, -0.072 and 0.197.3 for Zhanjiang. ③Considering all factors, the accuracy of the proposed methods was ranked in BP> limit learning machine> wavelet neural network. ④After simplification, the accuracy of the methods was ranked in BP0 (considering all factors)>BP1 (considering only daily highest temperature, lowest temperature, relative humidity and sunshine duration) >BP2 (considering only daily highest temperature, sunshine duration).【Conclusion】 ET0 calculation can be simplified by the BP algorithm based on the highest daily temperature and sunshine duration. |
Key words: ET0; neural network; Penman-Monteith; factor sensitivity analysis; model |
|
|