This article has been:Browse 1558Times Download 2382Times |
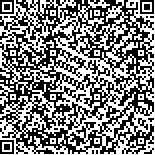 scan it! |
|
DOI:10.13522/j.cnki.ggps.2019121 |
|
Predicting Change in Water Availability for Jinghui Irrigation District Using Ensemble Empirical Mode Decomposition and Back Propagation Neural Network |
LI Wenqing, LIU Zhao, WANG Lixia, LI Qiang, WU Xiaohong
|
1.School of Water and Environment, Chang’an University, Xi’an 710054, China;2.Key Laboratory of Subsurface Hydrology and Ecological Effects in Arid Region of Ministry of Education, Chang’an University, Xi’an 710054, China; 3. Institute of Water and development, Chang’an University, Xi’an 710054, China; 4. College of Geological Engineering and Geomatics, Chang’ an University, Xi’an 710054, China; 5.The Administration Bureau of Jinghui Canal Irrigation Area, Sanyuan 713800, China
|
Abstract: |
【Background】Climate change and intensification of human activities have impacted hydrological cycling, resulting in a sharp decline in river inflow, frequent occurrence of extreme weathers and decline of groundwater table. The change in water resources has led to a great challenge to water resource management for both industry and agriculture, especially the massive irrigated areas in arid and semi-arid areas which rely on water diverted from rivers for irrigation. It is urgent to improve our understanding of potential change in water resource and irrigation demand to safeguard food production in these regions.【Objective】The inflow to the Jing river has been in decline and the available water for diverting to Jinhui irrigation district has been reduced as a result. The purpose of this paper is to analyze the factors affecting the change in water resources at the head of Jinghui Irrigation district in Zhangjiashan and predict its potential change. 【Method】A model based on the ensemble empirical mode decomposition (EEMD) and the back propagation (BP) artificial neural network was established. The measured hydrological data was used to train and correct the model prior to being used to predict runoff and sediment yield in the next 10 years from 2018 to 2027 at Zhangjiashan. In the meantime, we also analyzed the available surface water resources for the irrigation district.【Result】①The combination of the EEMD and the BP neural network could take the most of each to predict the nonstationary time series of the hydrological data. ②The change in water runoff and sediment yield at the head of the canal of the irrigation district was relatively consistent and simultaneous, both rising first followed by a decline in the next 10 years from 2018 to 2027. ③The average annual runoff will be approximately 1.186 billion m3, slightly higher than the average over 1996—2017, while the average annual sediment yield will be about 100 million tones, slightly lower than the average over 1996—2017. ④The available water resources at Zhangjiashan from 2019 to 2027 will be relatively stable, approximately 779 million m3 annually, slightly higher than the average over 1996 to 2017, and can meet the demand of the irrigation district for water diversion at current level. But management is required to ensure enough water will be able in dry years.【Conclusion】The errors of the model were in acceptable range, and the predicted results matched well with the measured data. The EEMD-BP model is accurate and reliable for predicting annual runoff and sediment yield, and it thus provides an alternative to help improve efficient utilization and management of water resources so as to safeguard agricultural production in the studied irrigation district. |
Key words: water resources; annual runoff; forecasting; nature of runoff and sediment; irrigation district |
|
|