This article has been:Browse 1537Times Download 2376Times |
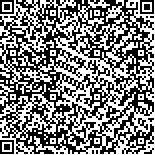 scan it! |
|
DOI:10.13522/j.cnki.ggps.2020056 |
|
Comparing the Performance of GPR, XGBoost and CatBoost Models for Calculating Reference Crop Evapotranspiration in Jiangxi Province |
LIU Xiaoqiang, DAI Zhiguang, WU Lifeng, ZHANG Fucang, DONG Jianhua, CHEN Zhiyue
|
1.College of water conservancy and ecological engineering, Nanchang Institute of Technology, Nanchang 330099, China; 2. Key Laboratory of Agricultural Soil and Water Engineering in Arid and Semiarid Areas, Ministry of Education, Northwest A&F University, Yangling 712100, China; 3. Faculty of Agriculture and Food,Kunming University of Science and Technology,Kunming 650500, China; 4. College of Hydrology and Water Resources, Hohai University, Nanjing 210098, China
|
Abstract: |
【Background】Alternate drought and waterlogging increasingly occurring in Jiangxi province means that rational irrigation strategies are required to safeguard its agricultural production.【Objective】The objective of this paper is to select a suitable machine learning model to calculate reference crop evapotranspiration across the province.【Method】Meteorological data - including daily maximum (Tmax) and minimum (Tmin) ambient temperature, global solar radiation, extra-terrestrial solar radiation(Rs), relative humidity (RH) and 2m-height wind speed (U2) - were measured from 2001 to 2015 at 15 stations across the province; they were then used to train and test three models: The gaussian process regression (GPR), the extreme gradient boosting (XGBoost), and the gradient boosting with categorical features support (CatBoost). We compared accuracy with empirical model for estimating the reference evapotranspiration.【Result】The meteorological factors that impacted the accuracy of the machine learning model for estimating ET0 was ranked in the descending order as follows based on their significance: Rs>Tmax>Tmin>RH>U2. Models using Tmax, Tmin, Rs and U2 gave the most accurate ET0 estimate with RMSE<0.2 mm/d. All three models have a good applicability by using limited meteorological data, and are superior to the traditional empirical model. In particular, GPR and CatBoost were more accurate, and GPR was most stable.【Conclusion】In terms of complexity, accuracy and stability, GPR was the most suitable model for estimating reference crop evapotranspiration in Jiangxi province. |
Key words: reference crop evapotranspiration; gaussian process regression; extreme gradient boosting; gradient boosting with categorical features support; empirical model |
|
|