This article has been:Browse 1796Times Download 2120Times |
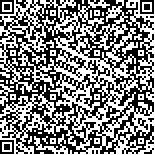 scan it! |
|
DOI:10.13522/j.cnki.ggps.2020531 |
|
Predicting Droughts in Growth Season of Spring Maize with the Wavelet Neural Networks using Particle Swarm Optimization Training |
CAO Xiujia, GU Jian, MA Ningning, LIU Yongqi, WANG Zihao, YIN Guanghua
|
1. Institute of Applied Ecology, Chinese Academy of Sciences, Shenyang 110016, China; 2. University of Chinese Academy of Sciences, Beijing 100049, China; 3. Tillage and Cultivation Research Institute, Liaoning Academy of Agricultural Science,Shenyang 110116, China; 4. Shenyang Agricultural University, Shenyang 110016, China
|
Abstract: |
【Background】 Drought is the most common natural hazard occurring more frequently over the recent years, due to climate change, and could have calamitous impact on agriculture. In northeast China, damage caused by long-lasting and frequent droughts is especially severe and has been in increase over the past years. Forecasting drought occurrence in plant growth season is hence important to safeguard agricultural production.【Objective】This paper is to present and test a model for drought forecast in efforts to offer a guidance to water-saving irrigation for spring maize in the northeast of China.【Method】The Pearson correlation coefficient method was used to select the factors that impact the drought index most, based on daily meteorological data measured from 1965-2019 at Fumeng county in Fuxin City of Liaoning province. We then forecasted the crop water deficit index at different growth stages with the wavelet neural network model using the particle swarm optimization.【Result】The root mean square error (RMSE) of the drought forecasted by the model at sowing-seedling, seedling-joining, jointing-tasseling, tasseling-milking, and milking-maturity stages was 0.041 9, 0.017 4, 0.048 1, 0.029 7 and 0.042 1 respectively, and their associated determination coefficient was 0.840 2, 0.985 3, 0.899 0, 0.957 5 and 0.917 7 respectively. These were consistent with the ground-truth data, proving that the model is suitable for drought forecast in these areas. There were no or only mild droughts in the sowing-seedling stage, but moderate or even extreme drought may occur during the seedling-jointing stage. As the crop grew, the occurrence of severe drought became increasingly unlikely especially in the milking-maturity stage, although mild drought might still occur in the jointing-tasseling and tasseling-milking stages.【Conclusion】The spring maize in the studied area is most prone to drought during the seedling-jointing stage, and our results are of significance to precision irrigation, mitigating detrimental impact of droughts on agricultural production. |
Key words: drought forecast wavelet neural network; spring maize; crop water deficit index |
|
|