This article has been:Browse 1179Times Download 2052Times |
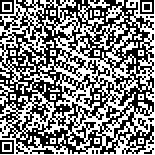 scan it! |
|
DOI:10.13522/j.cnki.ggps.2022141 |
|
Using Unmanned Aerial Vehicle to Evaluate the Effect of Nitrogen Fertilization on Winter Wheat Yield |
DING Fan, CHEN Zhen, LI Changchun, CHENG Qian, FEI Shuaipeng, LI Jingbo, XU Honggang, LI Zongpeng
|
1. Henan Polytechnic University, Jiaozuo 454003, China; 2. Farmland Irrigation Research Institute,
Chinese Academy of Agricultural Sciences, Xinxiang 453002, China; 3. Henan Agricultural University, Zhengzhou 450002, China
|
Abstract: |
【Objective】Precision agriculture requires a quick and accurate evaluation of the response of yield to managements at different growing stages, which is challenging at the large scales because of the heterogeneity of plants and soils. Remote sensing technologies can plug this gap, and the purpose of this paper is to investigate the feasibility of using unmanned aerial vehicle (UAV) to evaluate the variation in winter wheat yield in response to change in nitrogen fertilization.【Method】Canopy spectral information of winter wheat under different nitrogen fertilizations was measured using a multispectral sensor mounted on a UAV at heading, flowering and filling stages, from which we extracted the vegetation indexes. Stepwise regression and random forest models were used to screen the optimal indexes for estimating the wheat yield.【Result】Combination of green (G), blue (B), modified soil-adjusted vegetation index 2 (MSAVI2) and soil-adjusted vegetation index (SAVI) at heading stage works best to predict the yield with low AIC (Akaike Information Criterion) and R2=0.65. Combination of excess green (ExG), near infrared (NIR), normalized difference index (NDI), B and modified soil-adjusted vegetation index 2 (MSAVI2) at flowering stage is the best when using the random forest algorithm to predict the yield with low AIC and R2=0.71. The random forest regression model using all vegetation features at filling stages gave the most accurate prediction of the yield with R2=0.76, compared with R2=0.76 when using G, B, ExG, excess red (ExR), normalized difference vegetation index (NDVI), ratio vegetation index (RVI) and normalized difference vegetation index (TNDVI) only. Prediction using B at heading stage, ExG at flowering stage, NIR at filling stage, NDI at filling stage, and excess green-excess red (EXG-EXR) at filling stage predicted a yield with R2=0.76. 【Conclusion】Combination of the vegetation indexes measured using multispectral sensors at different growing stages can predict the ultimate winter wheat yield, but the accuracy varies. Best results are achievable using a combination of different indexes measured at different growing stages. |
Key words: multispectral; vegetation index; stepwise regression; random forest; yield prediction |
|
|