This article has been:Browse 419Times Download 1509Times |
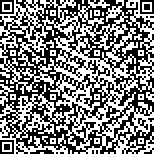 scan it! |
|
DOI:10.13522/j.cnki.ggps.2024218 |
|
Predicting spring maize yield using UAV remote sensing |
MA Shijiao, FANG Chengtai, ZHAO Jinghua, LIU Feng, YANG Tingrui, YUAN Ruxin
|
1. College of Water Conservancy and Civil Engineering, Xinjiang Agricultural University, Urumqi 830052, China
2. Xinjiang Key Laboratory of Hydraulic Engineering Security and Water Disasters Prevention, Urumqi 830052, China
3. Corps Soil and Water Conservation and Water Resources Development Center, Urumqi 830002, China
|
Abstract: |
【Objective】Predicting potential crop yield is critical not only to policy making but also to improving agricultural management to safeguard food production. In this paper, we investigate the feasibility of using unmanned aerial vehicles to predict crop yields.【Method】Spring maize was used as the model plant. The field experiment consisted of five soil moisture treatments by irrigating 50% (W1), 75% (W2), 100% (W3), 125% (W4) and 150% (W5) of estimated evapotranspiration. Multispectral images captured by an unmanned aerial vehicle (UAV) were used to construct the vegetation indices, and the pearson’s correlation coefficient method was used to screen the input variables for the yield prediction models. Partial least squares (PLS), random forest regression (RF), and particle swarm optimization (PSO) were used to develop and optimize yield prediction models based on the UAV images obtained at the nodulation, tasseling and filling stages of the maize growth. The grain yield was predicted using both single vegetation index and multi-vegetation indices, with the most accurate model used to generate a yield map of the studied area.【Result】Models using multiple vegetation indices were more accurate than models using single vegetation index. Among all models, the PSO-optimized random forest (PSO-RF) model was most accurate during the tasseling stage. Compared with the measured data, the PSO-RF model based on NDVI achieved an R2 of 0.685, RMSE of 1 792.71 kg/hm2, and RPD of 1.764, when using single index, while when using multi-indices, it achieved an R2 of 0.806, RMSE of 1 485.88 kg/hm2, and RPD of 2.032. The W3 produced the highest grain yield (19 845.25 kg/hm2), while the W1 resulted in the least yield (12 054.52 kg/hm2).【Conclusion】Multispectral UAV imagery combined with PSO-optimized random forest models offers a robust and accurate method for crop yield prediction. This method can serve as a valuable tool to improve agricultural management and optimize resource allocation. |
Key words: drone; spring corn; yield; vegetation indices; male extraction period; random forest regression |
|
|