This article has been:Browse 363Times Download 791Times |
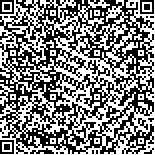 scan it! |
|
DOI:10.13522/j.cnki.ggps.2024066 |
|
Mapping soil salinity in irrigated areas using hyperspectral UAV imagery |
ZHOU Shixun, YIN Juan, WANG Juntao, CHANG Buhui, YANG Zhen
|
1. School of Civil and Hydraulic Enigineering, Ningxia University, Yinchuan 750021, China;
2. Yellow River Institute of Hydraulic Research, Zhengzhou 450045, China
|
Abstract: |
【Objective】Soil salinization induced by poor irrigation management poses a significant challenge to irrigated agriculture, reducing soil productivity and crop yields. Estimating soil salinity and its spatial distribution in irrigated areas can help improve soil and irrigation management. The objective of this paper is to use hyperspectral inversion techniques and a develop model to accurately estimate soil salinity and its distribution in the Hetao Irrigation District. 【Method】The experiment was conducted in the Shenwu Irrigation Area, where spectral reflectance and salinity data were measured and collected from 253 soil samples. Fifteen spectral transformations were applied to improve the correlation between hyperspectral data and soil salinity. Four models, including multiple linear stepwise regression (MLSR), partial least squares regression (PLSR), support vector machine regression (SVR), and backpropagation neural network (BPNN), were evaluated for their accuracy to estimate soil salinity. The most accurate model was then integrated with GIS to map soil salinity across the region.【Result】① Soil spectral reflectance increased with soil salinity, and spectral transformations significantly improved the correlation between hyperspectral data and soil salinity. ② Among the four models we compared, the BPNN model proved to be most accurate and stable. The optimal spectral transformation was the first derivative of the reciprocal logarithm of transformed reflectance data (represented by R), that is, lg(1/R)]'. This model achieved a determination coefficient of 0.825 and a root mean square error of 2.254 g/kg. ③ Integrating the BPNN model with GIS enabled estimation of spatial variation of soil salinity. Validation against ground-truth data revealed spatial pattern in soil salinity distribution, with high soil salinity found in the southeast, west and north, and severe soil salinization found in areas adjacent to the lake.【Conclusion】The BPNN model using [lg(1/R)]' we developed is accurate and reliable for estimating soil salinity using hyperspectral data. Combined with GIS, it facilitates accurate mapping of soil salinization in irrigated areas, offering valuable insights for salinity monitoring and sustainable management in the Hetao Irrigation District and similar regions. |
Key words: soil salinization; hyperspectral; spectral transformation; inversion model; spatial distribution |
|
|