This article has been:Browse 469Times Download 1630Times |
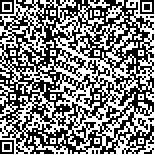 scan it! |
|
DOI:10.13522/j.cnki.ggps.2024049 |
|
Advances and applications of empirical mode decomposition and its variants in hydrology: A review |
CHEN Yunfei, LIU Zuyu, LIU Xiuhua, HE Junqi, ZHENG Ce, MA Yandong
|
1. School of Water and Environmental, Chang’an University, Xi’an 710054, China;
2. Key Laboratory of Subsurface Hydrology and Ecological Effects in Arid Region of Ministry of Education, Xi’an 710054, China;
3. Key Laboratory of Ecological Hydrology and Water Security in Arid Areas, Ministry of Water Resources, Xi’an 710054, China;
4. Shaanxi Academy of Forestry, Xi’an 710082, China
|
Abstract: |
Hydrological series are influenced by climate change, ecological succession, and human activities, containing complex, multi-layered, and interactive information that reflects highly non-linear and non-stationary characteristics. Effectively extracting and analyzing hidden information, while understanding temporal variation in hydrological data, remains a challenge. Empirical Mode Decomposition (EMD) has garnered increasing attention due to its ability to analyze non-linear and non-stationary data. This paper reviews the theory and application of EMD in hydrology, including its advantages and limitations. The review explores extensions and adaptations of EMD aimed at improving hydrological sequence analysis. We provide a comprehensive overview of the fundamental theory, methodological characteristics, and current challenges of EMD, covering five EMD-based methods: Hilbert-Huang Transform (HHT), Ensemble Empirical Mode Decomposition (EEMD), Multivariate Empirical Mode Decomposition (MEMD), Extreme Point Symmetric Mode Decomposition (ESMD), and Variational Mode Decomposition (VMD). The pros and cons of each method are analyzed. Additionally, we review the progress of EMD and its variants, particularly in the context of multi-spatiotemporal scale analysis, trend detection, and predictive modeling of hydrological series. Key insights are drawn from the use of EMD in detecting trends, identifying temporal variation features, and improving model predictions for hydrological data across different spatial and temporal scales. Despite the advancements in application of EMD and its variants in hydrology, challenges remain, including issues related to method robustness and efficiency in handling large-scale datasets. We conclude by offering recommendations for future research, including advancing EMD theory and enhancing techniques for analyzing hydrological variability at multi-temporal and multi-spatial scales under changing environmental conditions. |
Key words: data mining; multi-spatiotemporal scale analysis; hydrological variability analysis; trend tests; hydrologic forecasting |
|
|