This article has been:Browse 1727Times Download 3358Times |
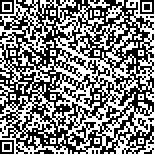 scan it! |
|
DOI:10.13522/j.cnki.ggps.20170058 |
|
Predicting the Probability of Precipitation with Meteorological Uncertainties in Consideration |
XING Zhenxiang, ZHANG Han, FU Qiang, GONG Xinglong, LI Heng
|
1. School of Water Conservancy and Civil Engineering, Northeast Agricultural University, Harbin 150030, China;2. The Key Laboratory of Efficient Utilization of Agricultural Water Resources of Ministry of Agriculture, Harbin 150030, China
|
Abstract: |
【Objective】The amount of precipitation is closely related to the change in meteorological factors, while the uncertainty of the meteorological factors affects the accuracy of precipitation forecast. This paper analyzed how to improve the accuracy of precipitation prediction considering uncertainty of the meteorological factors. 【Method】 Based on the Bayesian probabilistic forecasting system (BFS), a radial artificial neural network (RBF-ANN) was proposed to describe the relationship between the monthly precipitation and the main meteorological factors including evaporation, mean temperature and relative temperature for Youyi Farm in Sanjiang Plain. The above relationship was taken as a likelihood function of BFS, and the posterior information based on observed rainfall was used in the Bayesian modification of the pre-information of the main influencing factors. The posterior density of evaporation, mean temperature and relative temperature for each month was then obtained by the adaptive sampling algorithm in the Markov Chain Monte Carlo simulation method (AM-MCMC), from which the probability precipitation prediction model (IFU-PBF) was constructed. Using the IFU-PBF, we forecasted the mean precipitation and its confidence interval with specified probability. The uncertainty of the predicted precipitation was calculated.【Result】 Compared the deterministic prediction model RBF-ANN, the IFU-PBF improved the forecasted accuracy with the Nash efficiency coefficient increased by 3%, the mean square error reduced by 51% and the correlation coefficient increased by 2%. For predicting extreme precipitation, the relative error of the predicted the maximum value and minimum value using the proposed model increased by 55% and 24% respectively. 【Conclusion】 The IFU-PBF substantially improves prediction accuracy of the precipitation with the uncertainty of meteorological factors in consideration. The results provide a basis for water resource management and utilization. The probabilistic forecast is more appropriate by considering the stochastic nature of the precipitation and its associated factors. |
Key words: Bayesian Forecasting System; RBF-ANN; meteorological factors; uncertainty; probabilistic precipitation forecasts |
|
|