This article has been:Browse 1399Times Download 2967Times |
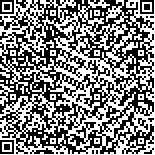 scan it! |
|
DOI:10.13522/j.cnki.ggps.2021038 |
|
Leaf Area Index of Summer Maize Estimated Using UAV-Based Multispectral Imageries |
XU Honggang, CHEN Zhen, CHENG Qian, LI Zongpeng, LI Peng, FAN Yongshen
|
1. Farmland Irrigation Research Institute of Chinese Academy of Agricultural Sciences/Henan Key Laboratory of
Water-saving Agriculture, Xinxiang 453002, China; 2. Henan Agricultural University, Zhengzhou 450000, China)
|
Abstract: |
【Background】Leaf area index (LAI) is an indicator of crop health and controls photosynthesis and transpiration of crops, however, its measurement is nontrivial. The traditional LAI measurement is point-based, time-consuming and laborious, and extrapolating the measured results to large scales could give rise to errors because of crop heterogeneity. The development in unmanned aerial vehicle (UAV) along with imagining technologies over the past decades had open a new avenue to reliably estimate LAI at large scales.【Objective】Taking summer maize as an example, the objective of this paper is to investigate experimentally the feasibility and accuracy of using multispectral UAV imageries to estimate LAI of the maize.【Method】The experiment was conducted in a maize field. Multispectral and thermal infrared imageries of the filed at different growth stages were taken by DJI M210V2 UAV equipped with Micasense Red Edge MX and ZenmuseXT2 dual photothermal imaging sensors. All imageries were first processed using the Pix4D software, and the results were then imported to ArcGIS to extract the thermal infrared canopy temperature (TC) and the multispectral vegetation index. Based on the ground-truth LAI data, we analyzed the correlation between LAI and the spectral data, from which an inversion model was established to estimate LAI using the vegetation index via three models: Multiple linear regression (MLR), support vector machine (SVM), and random forest (RF).【Result】①The multispectral vegetation index and TC were both correlated with the LAI at significant level (P<0.000 1), with the correlation coefficient being more than 0.5. ②The accuracy of the estimated LAI varied with the models and the crop growth stages. On average, the RF model was most accurate, and fitting the field-measured data at jointing, trumpet and silking stages showed that its associated R2 was 0.707, 0.834 and 0.849, respectively. The RMSE and NRMSE of the RF model were also smaller than those of the MLR and SVM models. ③Fusin TC improved the accuracy of all three models for predicting LAI at the jointing stage more than at the other two stages. As the crop grew, the promotion effect gradually decreased while the accuracy of the RF model with the thermal infrared TC integrated was improved though the improvement varied with the growth stage. This indicated that including TC was important to improve LAI estimation.【Conclusion】Multispectral vegetation index and TC are strongly correlated to maize leaves, and the RF model was more accurate than the MLR and SVM models to estimate LAI. In all three models we tested, including TC can improve their LAI estimation. Methods provided in this paper offer an easy and quick way to estimate crop LAI and have implications for precision agriculture. |
Key words: summer maize; UAV; multispectral vegetation index; thermal infrared image; LAI; inversion model |
|
|