This article has been:Browse 1693Times Download 2129Times |
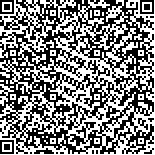 scan it! |
|
DOI:10.13522/j.cnki.ggps.2021073 |
|
Estimating Winter Wheat Yield Using UAV Remote Sensing Imageries and Stacking Method[ |
LI Zongpeng, LI Lianhao CHEN Zhen, CHENG Qian, XU Honggang, PANG Chaofan
|
1. Henan Agricultural University, Zhengzhou 450000, China;
2. Institute of Farmland Irrigation, Chinese Academy of Agricultural Sciences, Xinxiang 453002, China
|
Abstract: |
【Background and objective】Predicting potential crop yield based on crop traits at different growth stages is required in crop breeding and food safety management, but is challenging because of the spatial heterogeneity of crop traits and yield. The traditional yield prediction method is point-based, which is tedious and laborious, and the rapid development in unmanned aerial vehicle (UAV) and remote sensing technologies has started to break this barrier. The aim of this paper is to investigate the applicability of UAV and remote sensing, as well as their accuracy in predicting potential crop yield.【Method】The experiment was conducted in a winter wheat field. Remote sensing imageries of the crop at heading, flowering and filling stages were taken using a multispectral camera mounted in a drone. Using the multispectral bands derived from the imageries, 14 cropping indexes postulated to affect wheat yield were calculated. Based on the optimized vegetation indexes, different primary models including MLR, PLSR, SVM and Cubist were established for each growth stage to predict the eventual yield. We compared and analyzed all models and reconstructed the MLR and Cubist models for each growth stage using the Stacking method.【Result】As the wheat grew, the correlation between the vegetation index and the wheat yield increased, peaking at the filling stage with a correlation coefficient of 0.67. Comparison of the four primary models revealed that the Cubist model using data at heading, flowering and filling stages was most accurate to predict the potential wheat yield. Reconstructing the primary models using the Stacking method improved the accuracy of all models, with the Cubist model being the most accurate.【Conclusion】This study proves that fusing the primary models using the Stacking method can significantly improve their accuracy for predicting wheat yield. The methods and results in this paper have implications for predicting yield of other crops. |
Key words: multispectral imageries; vegetation index; stacking; method |
|
|